Why Why Matters
As a Buddhist, I’ve long been drawn to the dance of cause and effect. The Book of Why by Judea Pearl gave that intuition a scientific backbone. More than a book on data, it’s a powerful reminder that asking “why” is essential—not just in science, but in how we live and make sense of the world.
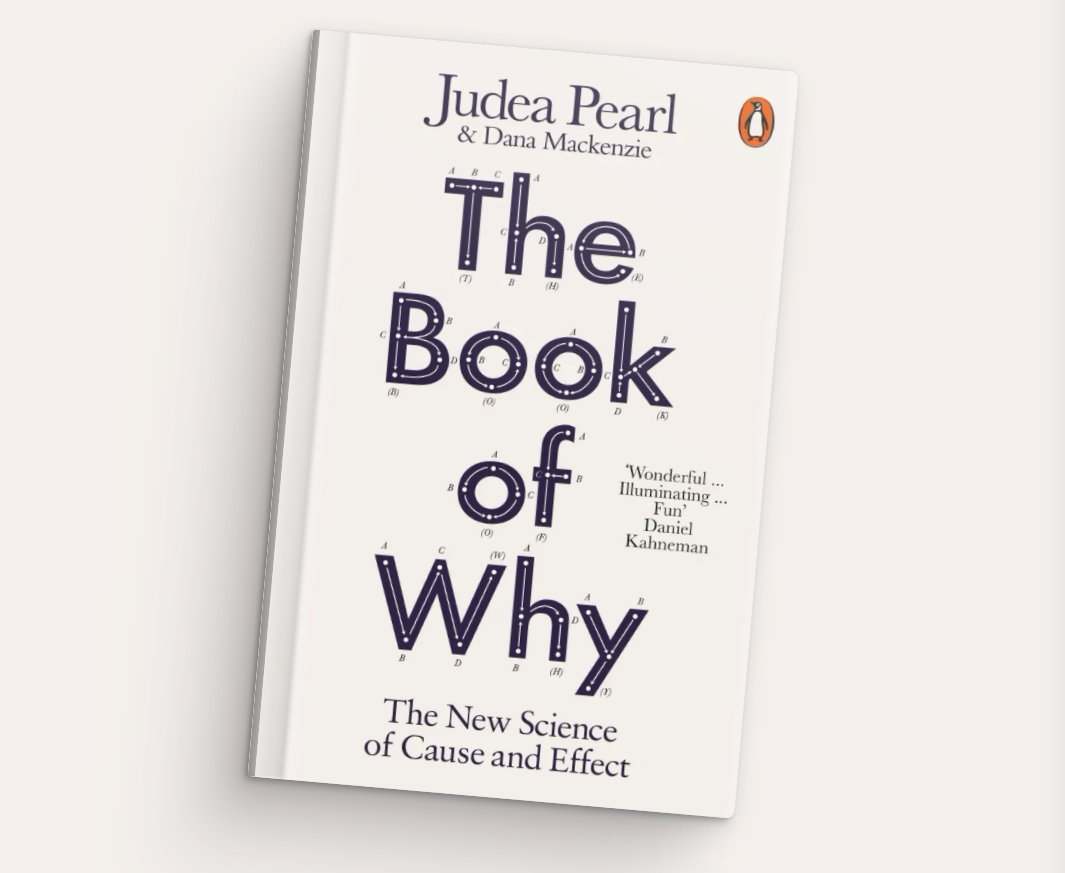
As a Buddhist, I've long been drawn to the idea of karma—not as a system of cosmic reward and punishment, but as a deep and intricate web of causes and effects. Causality, in this sense, isn’t just a philosophical curiosity. It’s the very structure of life unfolding: each moment, thought, and action linked to what came before and what comes after. So when I came across The Book of Why by Judea Pearl, I felt like I was encountering a scientific counterpart to something I’d been intuitively contemplating for years.
My connection to the book runs deeper than just intellectual curiosity. Years ago, I was acquainted with Marianne Pearl, a journalist whose husband, Daniel Pearl, was tragically kidnapped and murdered in Pakistan in 2002 while reporting for The Wall Street Journal. Judea Pearl is Daniel’s father. Knowing the immense personal loss behind his public persona adds another layer of resonance to this book. Judea Pearl is not just a celebrated computer scientist and philosopher of causality—he’s a man who has stared into the darkest questions of “why,” in both his personal and professional life.
Reading The Book of Why reminded me that causality isn't just a technical issue confined to data science or AI. It's a fundamental lens for understanding the world—and ourselves. Without it, we can describe what we see, but we can't grasp how things come to be. Whether we’re trying to cure a disease, make ethical decisions, or understand our own paths in life, the ability to ask “why” is what separates knowledge from wisdom, and insight from mere observation.
The Blind Spot in Modern Science
For decades, science—and especially data science—has been dazzlingly good at identifying patterns. We’ve built powerful models that can tell us that A is often followed by B. Correlation is the fuel of statistics, and in many cases, it’s enough to make useful predictions. But here's the problem: correlation doesn’t tell us why something happens. It doesn’t distinguish between coincidence, consequence, or common cause.
This blind spot isn't just academic. It has real-world consequences. Before scientists accepted that smoking causes lung cancer, we had decades of data showing a strong correlation between the two. But correlation alone wasn’t enough. Policymakers, researchers, and even the tobacco industry leaned on that epistemological gap: “Sure, smokers get cancer—but we can’t prove smoking causes it.” It wasn’t until causal reasoning entered the conversation that real change became possible.
Judea Pearl argues that science has long suffered from a self-imposed silence on causality. Classical statistics—by design—refused to ask “why”, focusing only on what could be observed and measured. The irony is striking: while everyday human beings constantly navigate the world using causal logic (“If I leave now, I’ll catch the train”), our most powerful scientific tools were deliberately avoiding those same questions.
Pearl doesn’t just point out the problem—he proposes a solution. And it begins with reintroducing causality to the heart of scientific thinking.
Climbing the Ladder of Causation
To reintroduce causality into science and logic, Pearl offers a deceptively simple but profoundly powerful framework: the Ladder of Causation. This ladder has three rungs, each representing a different level of reasoning:
- Association – Seeing patterns: “What goes with what?”
This is where most statistics and machine learning systems operate. It’s about observing that people who take aspirin tend to have fewer heart attacks, or that ice cream sales and drowning incidents rise together (without implying one causes the other). - Intervention – Doing things: “What happens if I do this?”
This is the realm of experimentation. It's the difference between observing that aspirin is associated with fewer heart attacks, and running a clinical trial to see what actually happens when people take it. Interventional thinking is at the heart of how we test hypotheses and evaluate policies. - Counterfactuals – Imagining alternatives: “What would have happened if…?”
This is the most human rung—and also the most powerful. It’s the domain of regret, moral responsibility, and imagination. “If I had left earlier, I wouldn’t have missed my flight.” It allows us to reason not only about what did happen, but what could have happened under different circumstances.
Most modern AI systems, even the most advanced, are stuck on the first rung. They can spot patterns across massive datasets but can’t reason about interventions or alternate realities. Pearl’s ladder shows us that true intelligence—human or artificial—requires the ability to climb.
What makes this framework so striking is how intuitive it feels. We live on all three rungs, often switching between them without noticing. Yet our machines, our algorithms, and much of our scientific thinking still live in the flatlands of correlation. Pearl gives us both the vocabulary and the tools to begin the climb.
Implications for AI and Human Thinking
Pearl’s Ladder of Causation doesn’t just offer a new way to think about science—it poses a fundamental challenge to how we build and evaluate intelligence, both human and artificial.
Today’s most celebrated AI systems—think recommendation engines, even self-driving cars—are astonishingly good at pattern recognition. They excel on the first rung of the ladder. They know what tends to happen, based on billions of data points. But they often stumble when asked the simplest causal questions. They can say, “People who bought X also bought Y,” but they can’t reliably answer, “What will happen if we stop recommending Y?” or “What would this customer have done if they hadn’t seen that ad?”
This isn’t just a technical gap—it’s a philosophical one. Real understanding requires moving beyond observation. As humans, we constantly act and imagine. We simulate possible futures, learn from hypotheticals, and reflect on alternate pasts. It’s what allows us to grow, to empathize, to regret. In short: it’s what makes us human.
Pearl argues that the future of AI depends on bridging this gap. To build machines that reason like us—or even with us—we need to give them causal models. Not just data, but an understanding of how the world works and how it could work differently. The goal isn’t just to build smarter machines, but to design systems that can explain themselves, justify their actions, and help us navigate uncertainty with greater clarity.
And beyond AI, this has deep implications for how we think about knowledge itself. We often assume that the more data we gather, the more we’ll understand. Pearl reminds us that understanding doesn’t come from more information—it comes from better questions. And the best questions always begin with “why.”
Reflections: Why This Book Changed the Way I Think
Reading The Book of Why didn’t just sharpen how I understand science or technology—it shifted something deeper. As someone already attuned to causality through Buddhism, I found Pearl’s work to be a kind of bridge: between logic and intuition, between computation and contemplation.
It reminded me that causality isn’t just a tool for academics or engineers. It’s a way of living. Every decision we make, every story we tell ourselves about our past, every hope we place in the future—they all rest on causal thinking. We want to understand what brought us here, and what could lead us somewhere else. Without that, we’re just floating in patterns, without direction or meaning.
Pearl gives us the vocabulary—and the courage—to reintroduce “why” into spaces that had forgotten it. Whether it’s evaluating health interventions, designing AI, or just trying to make sense of a personal turning point, the ability to ask why is both radical and grounding. You'll hear it a lot from me if you work with me.
In a world increasingly obsessed with speed, prediction, and optimization, The Book of Why is a quiet but powerful invitation to slow down and think more clearly. To choose understanding over automation. And to remember that causality is not just a question—it’s a path.